How Can We Help? Let’s Talk!
Andy Crestodina, Cofounder, Orbit Media
Digital marketing is a test of empathy
Andy gives a quick walk-through of how we use data-driven empathy to create a website you and your visitors love.
Hear me out, it won’t take long!
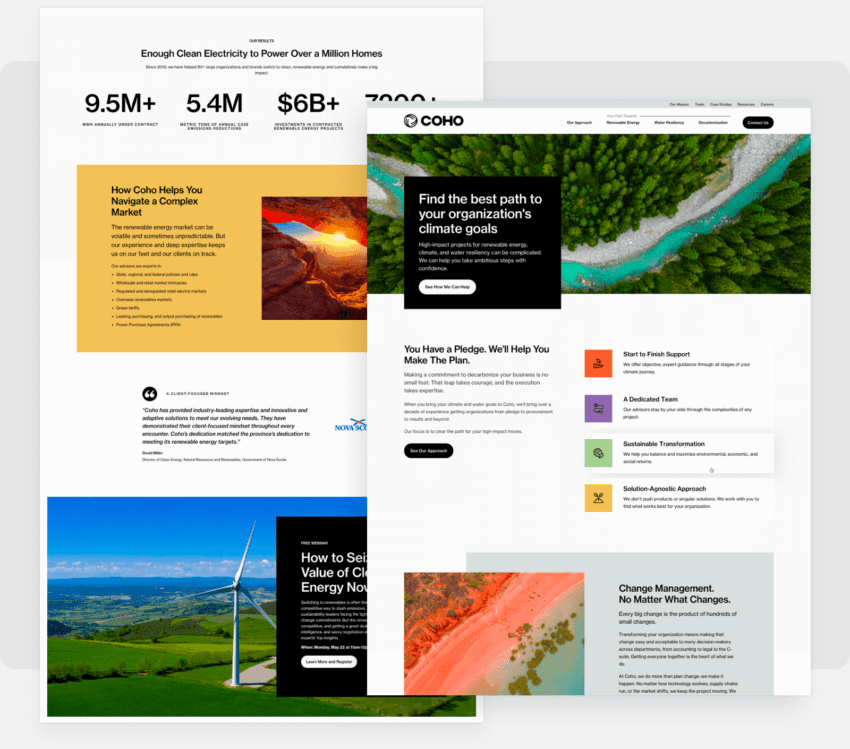
Partner with a digital marketing agency that’s designed and developed over 1000+ websites
We have worked with companies in your industry and have over 20 years of expertise in digital marketing, web design and development. Our digital marketing strategy and optimization services give us a perspective other creative agencies can’t offer.
- Research & competitive analysis
- UX & content marketing strategy
- Website content writing & migration
- Design & visual storytelling
- Website development & hosting
- Search engine optimization (SEO) and conversion optimization
We’re focused on creating exceptional work for clients
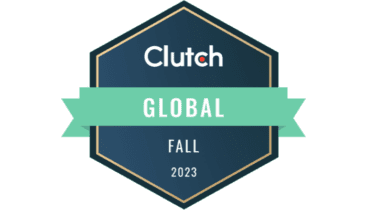
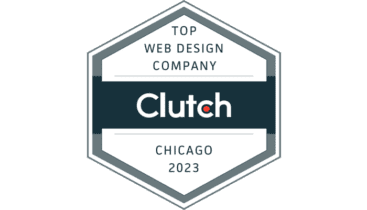
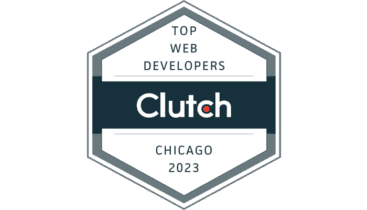
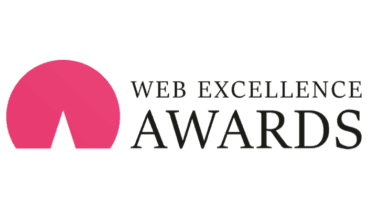
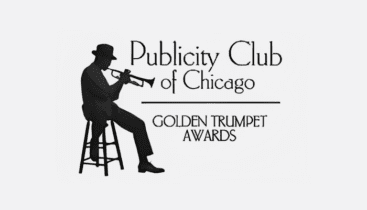
“Our website traffic doubled”
Tony, The Grieve Corporation, shares his Orbit experience
“Our (site) activity is double what it was the last 12 months. If you’re looking for a partner that’s going to help you get where you need to get to, Orbit’s the right group.”
Meet your web design and optimization team, the Orbiteers
A team of in-house web design, web development and optimization experts. Nothing is outsourced.
Our digital marketing services focus is on SEO driven website design, development and optimization. We have one purpose; build beautiful high-performing websites that generate leads.
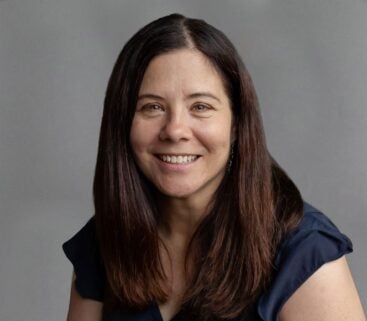
Digital Strategists
Align business and visitor goals with content, features, and measurable outcomes.
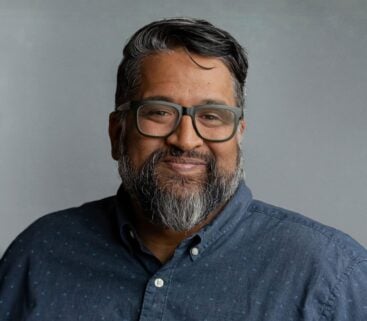
Project Managers
Monitor website project timelines and provides a dedicated communication resource.
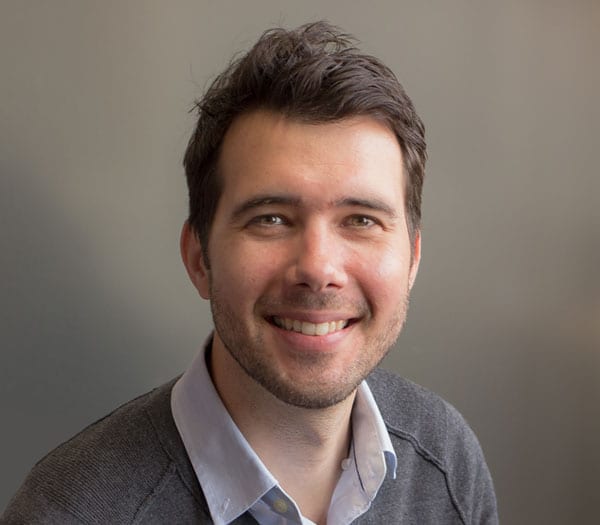
Web Designers
Collaborate with you to design a smooth experience and a visual extension of your brand.
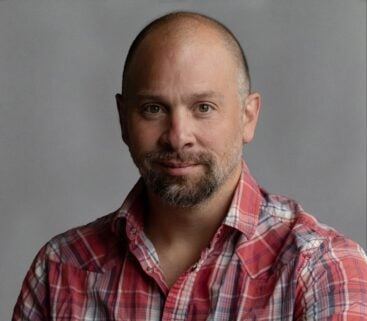
Content Specialists
Identify your goals, investigate assets, and write content that tells your story.
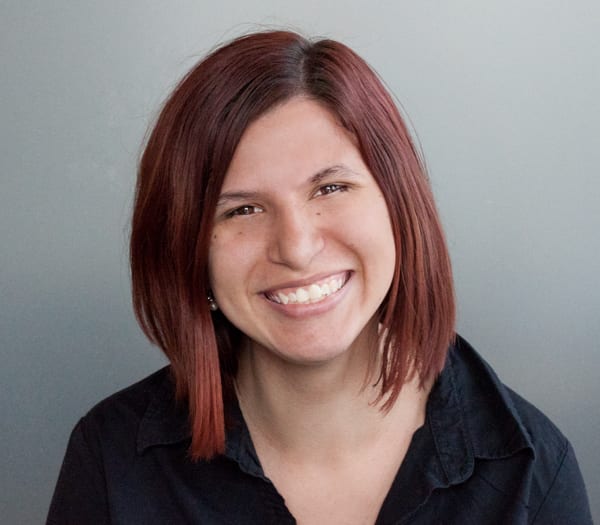
Web Developers
Build and integrate the features that ensure functionality and easy, efficient future updates.
“They have a great process and great team members.”
Diane Yetter, Founder of Sales Tax Institute
What it’s like working with the Orbit team
“We have a very data-intensive website. We were looking for better ways to make the information and resources that we offer to our users much more accessible.
Orbit has a great process and great team members.
Our business is not one that people want to learn about. The Orbit team wanted to understand who our customers were, what they’re looking for and what we offer.”
Optimize your website for higher conversions
Friction kills leads. Our SEO and CRO strategy enhance your website to improve leads and increase conversions.
Drive demand and increase leads with a website aligned to visitor psychology. If things are hard to find or your site is hard to navigate, people leave. Help visitors find what they want and what you want them to see. A conversion-oriented approach includes:
- Strategic sitemap & navigation
- Layouts with calls to action
- User experience (UX) analysis
- Conversion-oriented copy
When your website makes it easy for people to meet their goals (information), it’s far more likely that you’ll meet your goals (demand).
A data-driven web design and development company
A better website starts with research.
Curiosity is our starting point. We then launch into a thorough qualitative and quantitative investigation.
Our websites are built to rank. We perform keyword research, review analytics, use heat maps and eye-tracking technology. We identify current keyword rankings and capture new SEO opportunities for your brand.
At the same time, we interview you, the stakeholders and your audience. We perform competitive research. We listen and inquire, asking questions to understand your story.
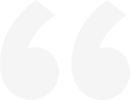
A digital web design agency that is 100% website focused
The fact that Orbit specializes in website design and development was a huge advantage in our experience. We’ve previously worked with agencies who said they could do 100 things and website design was just one of them. And it showed!
Update your messaging with powerful storytelling
Is your messaging out of date? Your website is an extension of your brand. It should reflect how you see yourself today.
Website design and content go hand-in-hand to tell your story. With insights gathered during our discovery, we craft content that captures your message, voice, and tone. Then we work with you to develop a beautiful website, a digital version of your brand.
- Develop brand conversion guides with messaging
- Ideate brand messaging and content strategy
- Gather and create assets, logos, iconography and brand standards
- Collaborate with you to design a site you love
By joining vision to story, websites appeal to your visitors and compel them to take action.
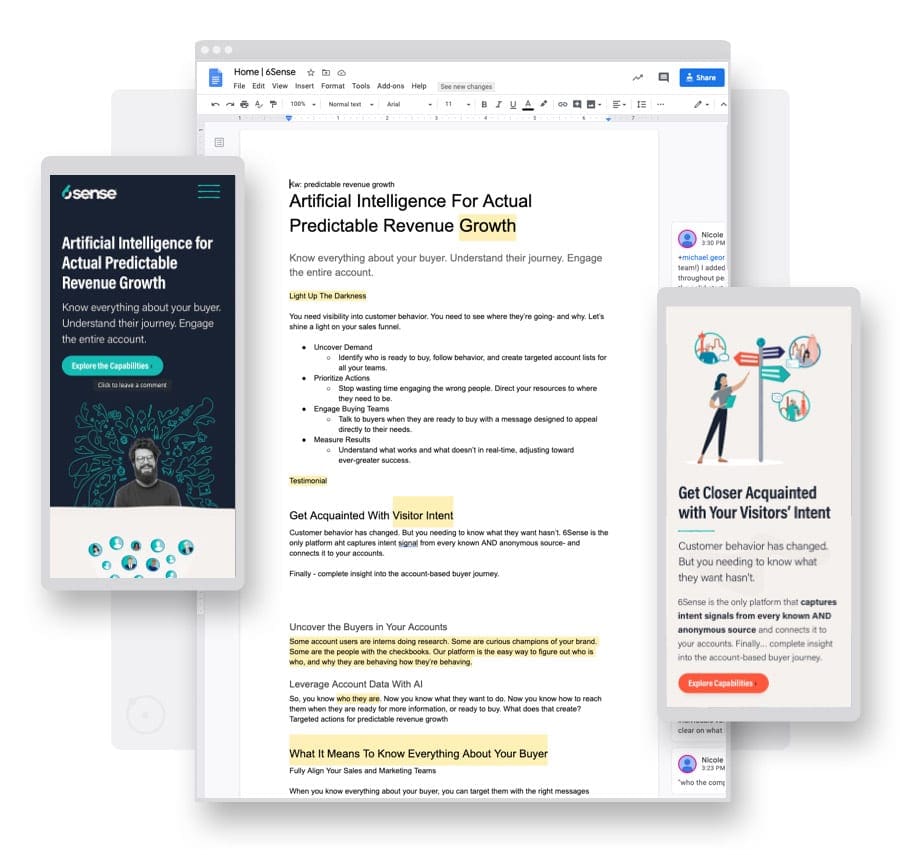
Edit any page in seconds
Website updates should be super simple.
Your new website will be developed specifically for your content and preferred processes. It will be easy for you on the backend and easy for your visitors on the frontend.
- Handle formatting, placement, permissions, and workflow
- Manage assets and automatically optimize images
- Transfer in content from other sources
“We will build your site so you can drive it yourself.” That line is from our website in 2001. That’s how long we’ve been developing easily managed websites.
Website design is a thousand tiny decisions
That’s why launching a website is just the beginning.
Each decision has a specific impact. Each impact has a measurable result. Each result can be improved.
- Gather accurate data through careful set-up of filters, goals, and funnels.
- Manage tracking codes from anywhere using Google Tag Manager
- Analyze regularly-delivered reports and dashboards
- Respond to post-launch data to improve results
- Enhance and optimize to continue success
Digital marketing is based on data. We turn data into insights and insights into high-performing websites, designed to tell your story and create measurable results for your business.
What if I’m not 100% sure what I want?
That’s understandable. Priorities shift during the process.
What you need from your web design company changes throughout the process and over time. We know this from our direct experience with over 1000 projects and clients.
While your goals are ever-present, your focus will shift as the web design process helps you redefine objectives. That’s to be expected. We adapt.
- Visual design, beauty, and brand
- Easy management, functionality, results, and ROI
- Care, service, collaboration, and responsiveness
The journey might change, but the end result is always a website you love.
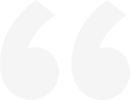
Website traffic tripled!
We more than tripled site traffic after our website redesign and we’ve been partnering with those web nerds ever since. Shopping cart abandonment is down, sales are up. HOT DOG!
What digital marketing services are right for you?
At the end of the day we want to make the internet a better place with websites that inform and convert. It’s our mission. We want you to love your website again.